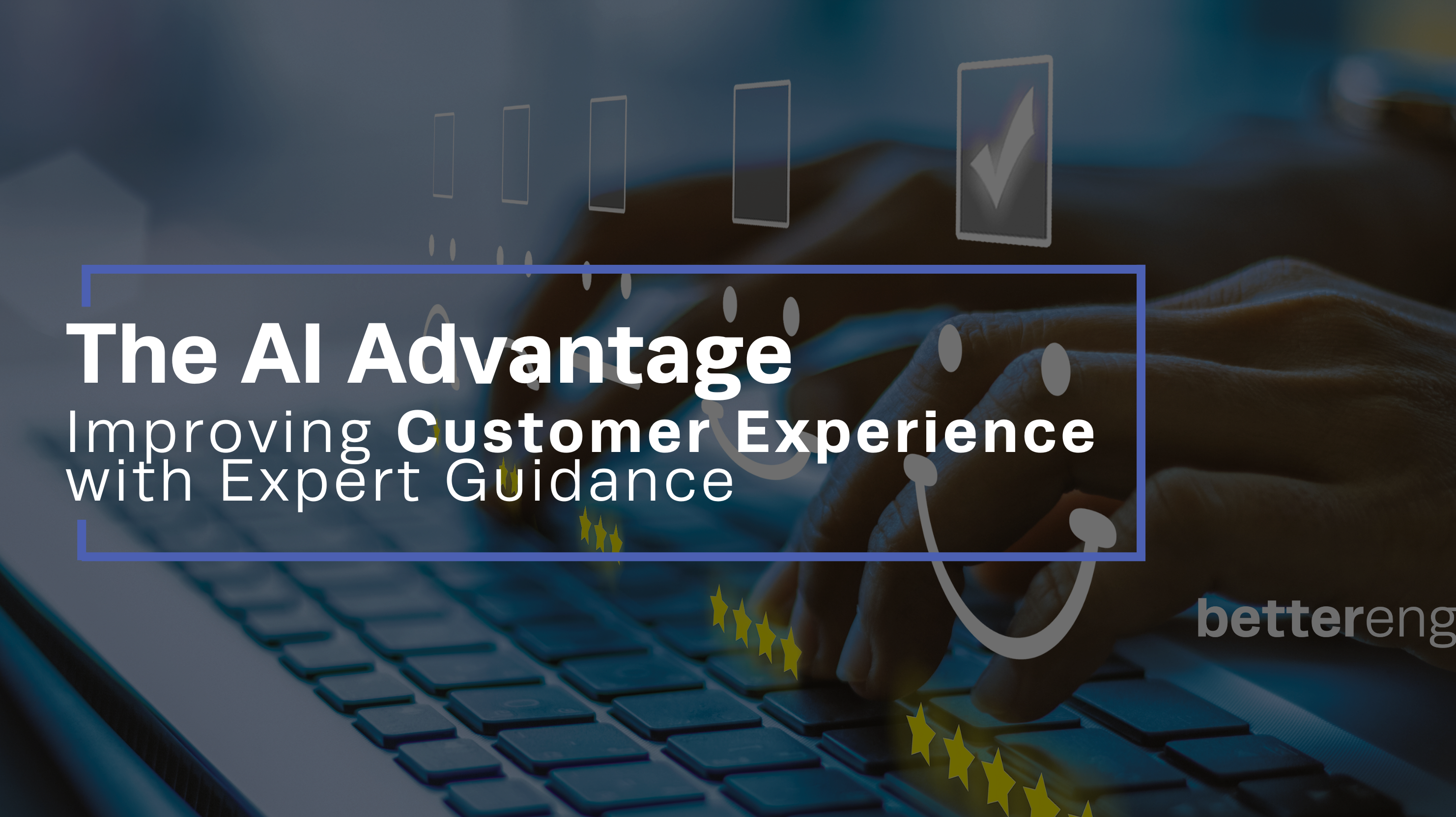
In today’s hyper-connected world, customer experience is everything. To build loyalty, increase conversions, and stay ahead of the competition, companies need to engage customers on a personal level. But not all businesses approach personalization in the same way. Some stick with outdated methods, making decisions based on static customer profiles, while others are stepping up by using AI to create more dynamic, tailored experiences.
In this blog post, we’ll explore 3 scenarios where AI can enhance customer experience, using fictional companies to bring these examples to life. Additionally, we’ll explain the role AI experts play in ensuring these technologies are implemented correctly and effectively.
Personalized Customer Journeys: A Story of Two Companies
Let’s look at two companies to see how different approaches to customer journeys play out—one clinging to traditional ways, and another using AI to enhance personalization. Their stories highlight the critical role AI plays in delivering personalized experiences and show why expert guidance, even for those managing AI themselves, can make all the difference.
Company Initech: The Traditional Approach
Initech relied on traditional, rule-based recommendations. Their marketing team manually segmented customers based on generic criteria like age and gender, sending the same promotional emails to everyone. The result? Low engagement, poor conversions, and customers who felt no real connection to the brand.
They gathered data but didn’t have the right tools to truly understand what their customers wanted. Despite a strong product lineup, their inability to offer personalized content led to high churn and low retention. When competitors started delivering more customized experiences, Initech found it hard to keep up.
Company Initrode: AI-Driven Personalization
Initrode took a contemporary approach by integrating AI into their customer journey strategy. With AI-powered analytics, they tracked real-time customer behavior, such as browsing history, purchase patterns, and social media activity. AI dynamically segmented their audience, offering personalized product recommendations and content that matched each customer’s unique preferences.
For example, if a customer often looked at eco-friendly products, AI would suggest sustainable goods tailored just for them. Additionally, if a customer transitioned from the website to a social channel, AI followed the journey, delivering real-time, context-appropriate messaging that maintained engagement across platforms.
Another key capability AI enabled was detecting when a customer revisited the pricing page. Upon sensing their return, AI adjusted the messaging through an A/B test, testing different content to optimize conversions. With this iterative, data-driven approach, Initrode achieved higher engagement, better conversions, and a more loyal customer base.
By automating the personalization and testing processes, the team focused on high-level strategy rather than manual data analysis. The best part? Initrode didn’t go it alone—they invested in fractional AI advisory services, tapping into expert advice on data optimization and the best algorithms to use. This ensured that every AI-driven customer interaction was optimized for success.
Why AI Guidance Matters
Even though Initrode was equipped with AI tools, the real game-changer was the guidance from an AI advisor. This advisor helped them choose the right machine learning models, optimize data usage, and refine their personalization strategy. Instead of spending a fortune on in-house AI experts, they accessed top-notch advice that ensured their AI investments paid off.
Without this expert input, companies like Initech often stumble—using inefficient algorithms, missing out on valuable data, or just not knowing where to start. Even a part-time AI advisor can provide the insights and course corrections that can make the difference between a good AI implementation and a great one.
Transforming Customer Support: Reactive vs. Predictive Approaches
AI isn’t just about offering personalized journeys —it’s about transforming the whole customer experience. According to a McKinsey & Company report, companies using AI-driven support saw a 15% to 20% increase in customer satisfaction scores due to faster problem resolution.
While some companies still rely on reactive strategies, waiting for problems to arise, others are using AI-driven predictive support to proactively resolve issues, enhance customer satisfaction, and reduce downtime. Let’s explore how Dunder Mifflin and Globex Corporation approached their customer support strategies.
Company Dunder Mifflin: The Reactive Approach
Dunder Mifflin relied on a traditional, reactive customer support model. Their team would respond only after customers encountered issues and reached out for help. The support system was manual and depended upon incoming support tickets, which often led to delays and frustration.
Without the ability to predict when an issue might arise, they couldn’t offer proactive solutions. As a result, customer satisfaction was low, and the company struggled with high churn. The team was overwhelmed with support requests, and by the time they addressed an issue, the damage to customer relationships had often already been done.
Company Globex Corporation: The AI-Powered Predictive Support Approach
In contrast, Globex Corporation took a completely different approach with AI-driven predictive support. Using machine learning models such as random forests, decision trees, and neural networks, the company could analyze patterns in data such as historical support tickets, system logs, user interactions, and sensor data from their products.
For example, they applied anomaly detection algorithms to spot irregular product behaviors that signaled early-stage failures. This analysis helped predict when customers would likely face issues, allowing proactive outreach before a malfunction impacted their experience.
The key data sources included:
- User Interaction Data: Monitoring customer behaviors and habits in real-time.
- Product Usage Logs: Data from sensors, error reports, and usage patterns.
- Historical Support Data: Trends from previous support cases that revealed patterns in product failures or customer issues.
By automating the process of spotting early warning signs, Globex Corporation was able to reduce downtime and keep customers happier, offering a proactive support experience.
The Need for Expert Insight
While Globex Corporation utilized AI algorithms, their success was magnified by expert guidance from an AI advisor. The advisor helped them:
- Select the right machine learning models, like random forests for feature importance and time-series forecasting models for understanding recurring customer patterns.
- Identify and integrate the most relevant data sources, ensuring the models were trained on high-quality, up-to-date information.
- Fine-tune their anomaly detection algorithms, ensuring accuracy and minimizing false positives.
- Create workflows that allow their support team to act on AI insights efficiently.
Without expert guidance, companies like Dunder Mifflin often struggle with inefficient AI deployments or underutilized data. In contrast, having fractional AI advisory services allows them to implement best practices, ensuring their AI investments produce real, measurable value.
Mastering Dynamic Pricing with AI
The third and last scenario is about dynamic pricing, a powerful tool that allows businesses to adjust prices in real-time based on demand, competition, and customer behavior. Let’s go back to companies Initech and Initrode and how they tackled this pricing strategy.
Company Initech: The Manual Pricing Approach
Initech relied on static pricing models. They reviewed prices every quarter based on past sales and market trends. This left them out of sync—sometimes overpricing when demand was low, or underpricing when demand was high. They missed out on revenue and couldn’t react quickly to market changes.
Because of their slow and outdated approach, Initech struggled to keep up with competitors who used more dynamic pricing strategies, often losing customers who found better deals elsewhere.
Company Initrode: The AI-Powered Dynamic Pricing Approach
In contrast, Initrode embraced AI to implement a dynamic pricing model. They used algorithms like regression models, random forests, and reinforcement learning to automatically adjust prices in real-time. Here’s how it worked:
- Demand Fluctuations: AI predicted spikes and dips in demand by analyzing both historical and real-time purchase data.
- Competitive Pricing: The system tracked competitor prices and adjusted their own to stay competitive.
- Customer Behavior: AI personalized prices for each customer based on browsing habits, past purchases, and shopping cart activity.
- Inventory Levels: Prices were lowered to clear out extra stock or raised when inventory was running low.
For these algorithms to work, Initrode pulled in data like:
- Historical sales figures
- Competitor prices from online platforms
- Customer interaction data (like browsing and cart activity)
- Supply chain and inventory levels
The Effort Behind Dynamic Pricing: Building a Dynamic Team
Initrode’s move to dynamic pricing didn’t happen overnight. They built a dedicated team of data scientists and engineers to create and refine the algorithm. Knowing the complexity involved, they also brought in outside experts for machine learning and data engineering through partners like BetterEngineer.
This specialized team handled:
- Cleaning up and integrating data to make sure the models were getting accurate information.
- Fine-tuning algorithms to find the right balance between staying competitive and boosting profitability.
- Continuously testing and adjusting the models to fit different market conditions
The Role of AI Guidance
AI guidance was key to their success. Initrode’s leadership engaged an AI advisor who provided fractional support to ensure they were using the right algorithms for their pricing models. The advisor helped:
- Select the right machine learning techniques, such as regression analysis for price elasticity and reinforcement learning for real-time adjustments.
- Suggested data sources for feeding accurate, timely data into the system.
- Designed Monitoring & Governance Procedures, helping Initrode avoid common pitfalls like over-adjusting prices or misinterpreting demand signals.
With expert AI guidance, Initrode was able to implement a dynamic pricing model that maximized revenue, improved customer satisfaction, and kept them ahead of their competitors—all without the need for a full-time AI team.
Transforming AI Potential into Tangible Success
We can conclude that the real game-changer isn’t just AI—it’s how well you put it to use. Having access to expert AI guidance ensures that companies not only choose the right tools and models but also maximize their potential for success. Whether you bring in part-time advisors or hire dedicated professionals, teaming up with AI experts can give you the strategic edge you need to stay ahead, innovate, and create personalized experiences that truly resonate with your customers.
Need to Boost Your Customer Experience?
BetterEngineer’s AI Readiness Assessment is designed to guide you every step of the way. With expert-led analysis, customized planning, and collaborative ideation sessions, we’ll help you optimize your current systems and create a clear, actionable roadmap toward your AI-powered future.
Let our seasoned professionals provide the personalized strategies and dedicated support your company needs to stay ahead of the competition. Contact us today to start your AI journey and achieve your business goals with confidence.